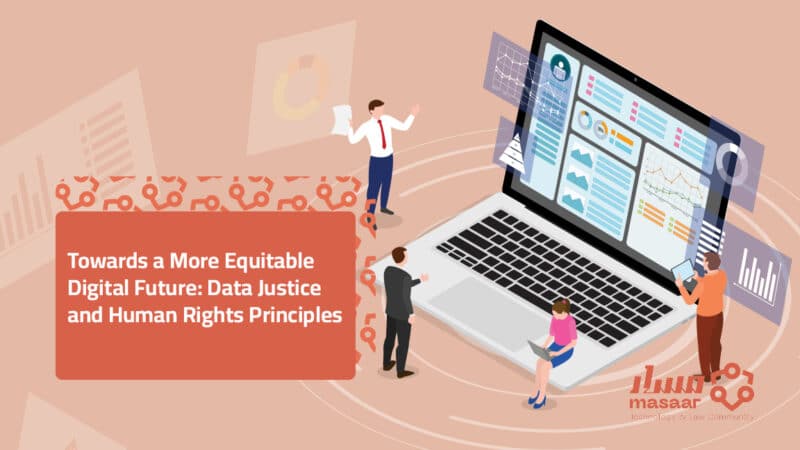
Introduction
Just as the concept of justice is essential for establishing the rule of law, data justice is vital for enhancing fairness in how people are represented, made visible, and treated based on their production of digital data. Embedding data justice in digital and human rights is critical for ensuring that the privacy, humanity, and dignity of all people are prioritized and advanced. Consequently, its fundamental benefits transcend mere data protection to encompass human rights and social justice.
Data justice has the potential to mitigate biases and inequalities, fostering fairness and social inclusion for vulnerable populations. Integrating data justice and human rights further enhances the transparency and accountability of data management and algorithmic fairness and safeguards individuals’ data. Consequently, there is a compelling need for a human rights-based approach to data justice that can spur optimal outcomes.
This paper aims to investigate the potential of integrating data justice principles with digital and human rights frameworks. It examines how this integration can facilitate the identification of biases based on ethnicity and gender within digital environments. By exploring this intersection, the paper aims to contribute to the promotion of a more just and equitable digital future.
The paper begins with a conceptual framework outlining the principles of data justice and their role in empowering individuals and safeguarding vulnerable populations. It then shifts to the main elements of data justice, focusing on equity in data access and collection, transparency and accountability, privacy and security, algorithmic fairness, participation and representation, and non-discrimination.
The paper also explores the intersection between data justice and human rights with case studies. Finally, it touches on the challenges experienced in implementing digital rights: technological challenges, regulatory challenges, and international disparities.
Data Justice in the MENA Region
On December 10, 1948, states agreed to embrace the Universal Deceleration of Human Rights, thereby assuming duties and obligations to safeguard, respect, and fulfill human rights. The Declaration encompasses rights such as the right to health, the right to science, and the right to privacy, which are crucial for safeguarding human dignity. However, efforts to ensure that the rights are met remain elusive and counterproductive, especially in the current digital environment that encounters many challenges.
Data justice can be traced to as early as 1890 in a Harvard Law Review article advocating for information privacy rights. In addition, in 1873, a government report has proposed that “fair information practices” should be embraced by organizations. Recent efforts towards data justice include the recent US Supreme Court rulings, including Carpenter v. United States, 2018; and, most notably, Dobbs v. Jackson Women’s Health Organization, 2022— which highlight the detrimental impact of weak privacy protections on marginalized and historically excluded groups. Violating privacy rights can also impede health equity by discouraging and demotivating people from taking part in research and even seeking healthcare.
The MENA region’s digital environment has been evolving for quite some time now. This evolution contributes to the evolution of corporate policies and legal frameworks. Tech firms in the region have been unable to ensure that the integrity of the digital environment is safeguarded. These firms over-moderate content, amplify misinformation, and engage in censorship during critical moments when reliable information-sharing is highly needed as illustrated by the most recent events of Israel and Palestine.
The MENA region experiences difficulties because various states, including Jordan, Iraq, and Tunisia, have laws that control online expression, media, and information sharing – these were introduced between 2022 and 2023. Other MENA states have also curtailed the freedom of expression by limiting free press coverage and expression, with journalists and citizens facing arrest and interrogation for criticizing their governments. Going forward this way, new digital policies and legislations will further influence the future digital environment impacting fundamental rights.
The concept of data justice encompasses various definitions provided by different authors and experts. According to some scholars, data justice is an approach concerned about means of collecting and disseminating data in an equal way without discriminating against historically marginalized communities. This definition implies that one of the core objectives of data justice is to advance human rights and reduce the harmful impacts of oppressive data practices.
The other definition is that data justice is a set of ideas that examines the representation of people or harm caused to them as a result of their data being visible to others. This definition means that data justice seeks to challenge and confront the existing structural biases, particularly the way we think about, collect, manage, organize, and even use our data. It also means that the data justice concept recognizes that data is not always neutral – and this means that there is often bias in how we collect, represent, make visible or hide data about individuals and communities.
In a period of rapid digital transformation characterized by processing large volumes of data, people’s rights can be violated, especially when data is not used appropriately or for intended purposes. To this end, data justice is so important because it illuminates the interactions of power dynamics between the actors, the politics that influence/drive data usage, the inclusion or exclusion of certain segments of society, and the interests of the parties involved (both individual and collective). Understanding how data is used is important, especially for vulnerable and marginalized groups, including refugees, who bear the brunt/burden of the most devastating impacts of dataveillance or surveillance.
Digital rights enable us to access, use, create, and even publish digital media. However, the marginalized groups often find themselves denied such rights. Notwithstanding, digital technologies influence the way fundamental rights in digital spaces are exercised, safeguarded, and violated. The rising number of technological advancements calls for new regulatory frameworks to ensure that our society remains responsive to the changing digital conditions. The 5G network continues to increase hyperconnectivity, and this has, over time, led to more data being collected and analyzed using devices from the Internet of Things and Edge Computing.
Due to the substantial amount of data, it becomes increasingly important for us to regulate the traffic of data and ensure that people’s digital rights are not violated. Therefore, we have to develop digital ethics (through data justice) to safeguard individuals and uphold their digital rights.
Section 1: Conceptual Framework of Data Justice
Data Justice Principles: Linnet Taylor’s definition of data justice is one of the most common definitions. Taylor defines data justice as fairness in how we treat people, make them visible, and treat them as we use and produce their digital data. Therefore, Taylor believes that data justice has three main pillars, as outlined below:
Visibility: Taylor argues that human beings have the right to privacy and to be represented with the right datasets. It means that in addition to the right to representation in data, we also have the right to informational privacy.
Technological (dis)engagement: it means that we are free to engage with technology as we want and be represented in commercial databases. Therefore, we have the discretion to engage with various technologies when we want. As we engage with them, we should consider the rights of vulnerable communities, such as refugees.
Non-discrimination: This is the power to identify and challenge biases in data use as well as the right to be free from discrimination. Many identity systems are structured to prioritize a standardized user profile. However, having such a profile disadvantages populations that do not fit into this stereotype. This principle emphasizes the need to get rid of bias associated with data-driven decision-making. Eliminating such bias helps to ensure that all people are considered as equal during decision-making on data use.
Apart from the three pillars, data justice is underpinned by a number of core principles as we highlight below:
Fairness: Ensuring fairness in how data users represent, treat, and render individuals visible during the production of digital data is vital for advancing data ethics.. This entails a procedural and substantive dimension. The substantive dimension entails committing to ensure just and equal distribution of costs and benefits. It also entails ensuring that people and groups are free from unfair bias, discrimination, as well as stigmatization. Besides, organizations or those who store data and use it should express fairness through prevention, mitigation or monitoring unwanted discrimination and bias. Certain authors look at fairness from the perspectives of equality, diversity, and inclusion. On the other hand, others emphasize the need to challenge or appeal data-related decisions. Overall, this principle emphasizes the need to have fair access to data and prevent harm.
Accountability: Accountability focuses on tracking the origin of data. It is defined as the data teams’ responsibility to manage and optimize their costs, resources, and processes. In addition, it entails being transparent regarding data costs, usage, ROI, fostering a cost-conscious team culture, and maintaining good data hygiene. Embedding accountability into decision-making and workflows can enable data teams to enhance their overall efficiency and have a positive contribution to the organization’s profits. Documented processes and procedures that aim to tackle data protection issues during an early stage are crucial for ensuring accountability within data operations.
Transparency: Besides being a compliance requirement, data transparency is a commitment to ethical practices that foster reliability and trust in data management. It involves various actions such as clear communication of data usage policies and ensuring that stakeholders comprehend the implications of data sharing. In an era where data is an influential and usually a sensitive asset, we cannot disregard the important role of transparency in maintaining the trustworthiness and integrity of those who handle it. The core benefits of data transparency are regulatory compliance, improved decision making, better public trust, enhanced innovation, enhanced accountability, faster problem identification and resolution, and encouragement of collaboration.
Inclusivity: Inclusive data is a pre-requisite for creating an equitable and fair society. It entails ensuring that all persons are heard, seen, and counted. It is also crucial for developing a society that values diversity and acknowledges all people’s unique experiences. The principles of inclusive data are governed by five key aspects: representation, disaggregation and intersectionality of all the identities, privacy, support, and the need for inclusive data to be obtained from multiple sources. Also, inclusive data serves as a foundation for informed decision-making while the objective is to utilize this data for structuring programs, policies, and strategies promoting equality and inclusivity.
Role in Protecting Vulnerable Populations:
Data justice systems help diagnose ethnic and gender biases. This helps to resolve disputes in a peaceful manner and protect vulnerable populations. Public health ethics frameworks recognize how data justice helps surveilled populations by ensuring that burdens and benefits are fairly distributed, reducing inequities and using the least intrusive surveillance forms required to meet public health goals. A data justice perspective further helps to evaluate data practices in terms of the existing social injustices. This is also critical to eliminate biases, enhance transparency and fairness, and advance data rights to empower marginalized groups while propagating social justice within data-driven contexts. Therefore, data justice is crucial to drive positive change in the use and collection of data. Communities are empowered to demand for their data rights, in decision-making, and challenge discriminatory practices – probably leading to more equitable outcomes for marginalized populations. In a nutshell, data justice contributes to reducing inequalities and biases, enhancing social inclusion and fairness for vulnerable populations.
Section 2: Key Aspects of Data Justice
Equity in Data Collection and Access:
Equity entails ensuring equal opportunities, fair treatment, and impartial access to resources and information for all. When this is adhered to, equitable access to data helps to ensure justice and inclusivity. Besides, it is important to acknowledge issues of bias, power, and discrimination when collecting, interpreting, and analyzing data. The domino effect is better data outcomes since it ensures that data quality improves, health and well-being are enhanced, and advancing long-term community partnership and sustainability. Summarily, equitable data access enhances community power, ensures inclusive data systems, promotes data access and sharing, safeguards privacy and confidentiality, and builds strong and inclusive data systems.
Strategies for ensuring fairness:
1- Enhancing Interpretability and Transparency of AI Models:
We should make AI models more interpretable and transparent to allow end-users and developers to understand why a specific decision was made, thus making it easier to unearth and rectify bias.
2- Embedding fairness into algorithms:
Employing strategies like adversarial training can bolster models against adversarial attacks aimed at introducing bias..
3- Promoting Diversity in Teams:
Organizations should further seek to dedicate resources for educating and raising awareness among developers, managers, and designers. It is also critical to ensure that there is a diverse team composition. An example is that firms can improve diversity within teams developing AI systems to ensure that they identify and address bias more effectively.
4- Adopting Clear Methodologies and Governance Frameworks:
Developing clear methodologies and adopting governance frameworks to revise AI pipelines in a more sustainable manner is important, including steps for detecting and mitigating bias.
5- Upholding Ethical Standards:
According to the Joint Commission, organizations should uphold ethical standards by documenting procedures and policies when it comes to data collection. This implies that organizations should communicate any changes or discrepancies with stakeholders, including those that relate to how fairly labels are applied. It is also critical for organizations to strive for equal representation when collecting data by getting rid of existing unfair representations in program materials and designs utilized with public audiences.
Transparency and Accountability:
In the current business landscape, transparent data shapes how organizations collect, handle, and use data. When organizations prioritize transparency, they instill trust and credibility with stakeholders and customers. Additionally, data transparency strengthens decision-making by fostering innovation and facilitating the adoption of the data-driven strategies embraced by organizational leaders.
By openly sharing information about how they gather and use data, organizations can foster trust with customers and stakeholders. Data transparency also fosters data-driven innovation and decision-making. Access to trustworthy data can enable businesses to make strategic decisions, spur growth, and provide competitive advantage. Transparency is also critical for enabling teams to explore insights and trends, which lay a foundation for developing new products or services.
Moreover, prioritizing data transparency enhances risk management by strengthening compliance and regulatory adherence that ensure businesses operate in legal boundaries. It is particularly important within strictly regulated sectors, including finance and healthcare. For example, pharmaceutical firms must comply with transparency regulations such as the Sunshine Act, which requires revealing financial relationships with healthcare providers.
Mechanisms to ensure accountability:
Clear data collection and storage protocols help to ensure that data is transparent and instill ethical management standards. Having specific guidelines can enable firms to collect and handle data that respects regulatory and privacy requirements.
Leveraging secure data infrastructure, including access and encryption controls, also improves data protection and reduces the risk of data breaches or unauthorized accesses. Such transparent approaches to data tracking instill trust among customers and enable firms to make informed decisions premised on ethically sourced and reliable data.
Also, educating stakeholders on how to ethically use data is an effective strategy for promoting data transparency. Firms can proactively engage with employees, customers, and partners to ensure they comprehend how to collect, store, and use data effectively and ethically.
For example, social media platforms can provide users with transparency reports and check-ups to empower them with knowledge and information on their data privacy settings. Addressing data security and privacy concerns is also a viable mechanism for overcoming resistance. Implementing strong data protection measures and adhering to relevant data protection laws such as the GDPR or CCPA demonstrates a firm’s commitment to safeguarding sensitive information..
Furthermore, implementing technology solutions can enhance data transparency and accountability in an organization. For instance, leveraging blockchain and decentralized technologies offers fixed records of data transactions, thus ensuring transparency while reducing the risk of manipulation or tampering. A more specific example is supply chain management platforms such as IBM Food Trust that use blockchain for tracking the movement of food products from farm to the dining tables, providing consumers transparent insight into their food’s quality and origin.
At the same time, data accessibility and visualization tools like interactive dashboards allow stakeholders to analyze and interpret complex datasets in an easy manner. They facilitate informed decision-making and transparency across several departments by empowering users with intuitive and clear data representations.
Privacy and Security:
Organizational data security standards help to safeguard sensitive and confidential information by preventing unauthorized use, disruption, access, disclosure, modification, or destruction of data. Failure to adhere to these standards and regulations can lead to legal penalties, action, and damage to the reputation of an organization. Therefore, Businesses must implement strong data security measures to safeguard their customers’ information and ensure compliance.
Data security’s main aim is to ensure that the data an organization collects, stores, transmits, or receives is secure. Compliance is also critical since organizations or data handlers should safeguard all data against all forms of threats. Given the different regulations and laws across regions and countries, organizations should ensure compliance with those relevant to their operational areas. For example, businesses operating in the EU should adhere to the GDPR and sector-specific regulations, including SOX or GLBA for financial services.
In the Middle East, the Personal Data Protection Law, Federal Decree Law No. 45 of 2021 regarding the Protection of Personal Data, is an integrated framework to ensure that information is confidential and safeguard people’s privacy in the UAE. Generally, general law provisions protect personal data and individual privacy in many Middle East and North African (MENA) jurisdictions. Other MENA states have also taken substantial strides in data security and privacy by implementing the UAE Data Protection Law (DPL), Saudi Arabia, Oman, and Bahrain’s Personal Data Protection Law.
Algorithmic Fairness:
AI bias can manifest in various forms, including selection, algorithmic, and measurement bias. Algorithmic bias is caused by limitations or errors in methods of collecting data, leading to skewed results. It is defined as the algorithmic decisions that systematically discriminate certain groups. Other experts also define it as the errors in a computer system that lead to unfair outcomes as a result of limited or skewed input data, exclusionary practices, or unfair algorithms during AI development.
Socio-technological factors are among the main causes of algorithmic biases. These include:
- Cultural, Economic, and Social Contexts: The design, deployment, and use of AI systems are influenced by these contexts, which can embed biases into the algorithms.
- Prejudice in design: including implicit biases of AI designers that can be transferred into a system’s behavior.
- Data bias: When the data used for training an AI system does not represent the entire population, the algorithm’s decisions can favor one group over others.
For example, hiring algorithms such as Amazon’s algorithm that once automated its recruitment process, ultimately favored males over females. The other example is facial recognition systems such as those used in smartphone unlocking and surveillance have been found to perform poorly with female and dark-skinned faces due to the lack of diversity within training datasets.
To mitigate algorithmic biases, several key strategies can be employed:
- Collecting Representative and Diverse Datasets:
This involves sourcing data from several demographics, ensuring fairness and inclusivity in representation.
- Exclusively Implementing Algorithms to Detect and Mitigate Bias:
Employing techniques such as fairness constraints, adversarial training, and bias audits can help to identify and rectify biases in models.
- Regular Monitoring of AI Systems Post-Deployment:
It is crucial to regularly monitor AI systems for biases post-deployment. Conducting frequent audits and establishing evaluation metrics can ensure that biases are identified and emerge over time or because of evolving datasets.
- Diverse Teams in AI Development:
Diverse teams working on algorithmic use in AI development should bring varied perspectives. This will help to identify and tackle biases in the design phase. Encouraging interdisciplinary collaboration instills a better understanding of potential biases.
Participation and Representation:
Diversity and inclusion are critical elements in machine learning and data science. Diverse representation offers numerous benefits, such as enhanced problem-solving, decision-making, and innovation. By leveraging various experiences, perspectives, and backgrounds, firms can unlock new insights and develop more inclusive solutions and products that cater to various users.
Diverse representation is also important to reduce the risk of biased algorithms and make sound decisions. For example, adequate representation of people of color in the training data of facial recognition systems can eliminate inherent biases, resulting in fair treatment and inclusion of all communities or individuals.
In addition, services and products developed by considering diverse users’ needs can lead to optimal solutions. For example, voice recognition systems that perfectly understand languages or accents other than English can ensure that non-native English speakers are included. This enables them to have access to the full range of benefits that the technology provides. Including diverse voices during the development process can lead to products that meet to various users’ needs.
Non-Discrimination:
Unconscious bias, usually unintentional and rooted in societal beliefs and norms, plays a critical role in discriminating and perpetuating the lack of diversity in machine learning and data science.
Discrimination can influence hiring decisions and make certain groups to be underrepresented. For example, certain organizations evaluate identical resumes differently based on factors such as ethnicity, race, or gender.
To tackle this issue, firms should implement strategies for mitigating unconscious biases in promotion and hiring processes. These include:
- Embracing blind recruitment practices: by detaching the identifying information from applications and resumes, focusing on qualifications and skills instead of personal characteristics.
- Implicit bias training: Provide training for hiring decision-makers and managers to generate awareness and counteract unconscious bias.
- Education and training: promote inclusion and diversity through equitable access to quality training and education programs. Creating opportunities for people from diverse backgrounds can help them succeed in these fields.
- Increasing diversity in STEM education: promote STEM programs in schools from a young age, offer financial support and scholarships, and develop inclusive curricula that celebrates diverse perspectives. This approach encourages diverse participation in STEM fields from an early stage.
- Internships, Apprenticeships, and Mentorship Programs: provide practical experience and support for people from underrepresented groups. These initiatives help bridge the gap between education and professional opportunities, fostering diversity in the workforce.
By implementing these strategies, organizations can reduce unconscious bias and promote greater diversity and inclusion in machine learning, data science, and related fields.
Section 3: Data Justice and Human Rights
Intersections with Human Rights:
A human-rights based analysis can benefit policymakers and the scientific community to advance human rights through data management practices. Such a framework has a shared set of norms and values across borders, defines responsibilities and rights of diverse actors in data sharing, examines the potential benefits and harms of data sharing and provides a framework for balancing various competing values.
The international human rights framework notably outlined in the Universal Declaration of Human Rights (UDHR) incorporates numerous principles embedded in the core international human rights treaties. The complex legislations, regulations, and professional codes applicable to data sharing, often share common features based on these international human rights principles. Ideally, human rights treaties usually highlight the obligations of governments to their citizens and to each other. This implies that a human-rights-based approach is all-inclusive for all persons/stakeholders who engage in the data life cycle.
This approach recognizes the government’s obligations to create legal structures for protecting those rights and holding those violating the rights accountable for their inappropriate actions. In addition, it entails access to information, access to justice, due process, and accountability for crime perpetrators. Human rights law further acknowledges community and individual rights.
According to international human rights law, besides individual rights, communities can also hold rights. Notably, community rights are usually linked with the indigenous people’s rights. However, they can also examine other disadvantaged communities’ rights. Enforcing community rights is quite similar to enforcing the individual rights and is characterized by due process, accountability, and transparency. Thus, applying human rights to data sharing/justice may not address all the issues associated with data use and sharing, but can ensure that we prioritize human rights during data management. This can help to ensure that data practices are accountable, transparent, non-discriminatory, and advance equity while minimizing any potential harm to minorities and other individuals.
Case Studies:
Case Study 1:
Bias in Online Recruitment: Amazon, an online retailer, faced significant gender bias issues with its hiring algorithm. Despite having a workforce composed of 60% males, a striking 74% of managerial positions were held by men. Recently, Amazon discontinued the use of its hiring algorithm after realizing it entrenched gender bias. Resume data was used by engineers to develop algorithms. The data was submitted to Amazon for more than one decade, and was mainly from white males. Instead of focusing on relevant skill sets, the algorithm identified certain word patterns within resumes. In addition, the data was compared against Amazon’s predominantly male engineering department to ascertain the suitability of an applicant. Consequently, the AI software was biased against any resume with the word “women’s” and downgraded the resumes of women who were attending women’s colleges, leading to gender discrimination in the hiring process.
Case Study 2:
Bias inherent in Facial Recognition Technology: According to Joy Buolamwini, algorithms powering three commercial software systems were unable to recognize darker-skinned complexions. Generally, many facial recognition training datasets are more than 75% male and 80% white. In a situation where the image/photo had a white man, the software becomes 99% accurate in identifying the person as male. These findings influenced Microsoft and IBM to commit to improving their recognition software’s accuracy for darker skinned faces.
Case Study 3:
Criminal Justice Algorithms: Judges use the COMPAS (Correctional Offender Management Profiling for Alternative Sanctions) algorithm to predict whether to detain or release defendants on bail pending trial. However, a report from ProPublica found it biased against blacks/African-Americans. The algorithm often assigns a risk score to the likelihood of a defendant to commit a future offense, depending on the voluminous data available on defendant demographics, arrest records, as well as other variables.
Unlike whites who were equally likely to re-offend, the report stated that African Americans were likelier to be assigned a higher-risk score, leading to elongated periods of detention as they awaited trial. Thus, this illustrates data practices that affect human rights despite Northpointe, organization that sells the algorithm’s outputs, providing evidence to refute such claims and believes that inappropriate metrics are being utilized to evaluate fairness within the product.
Whereas these biases are not exhaustive, they identify how these issues are empirical realities rather than mere theoretical concerns. They also denote how these outcomes emanate, and in some cases, without the algorithm operators or creators’ malicious intent.
Analytical Approach:
Data justice has played a critical role in understanding the power dynamics in data management and use. It has particularly been crucial for highlighting the actors involved, politics behind data use, inclusion or exclusion of groups, and parties’ collective and individual interests. Datafication has increasingly been acknowledged because of more knowledge on the use of digital data. It is common among vulnerable groups, such as refugees, who have borne the main burden of dataveillance. Thus, data justice brings about social justice and safeguards those who are vulnerable and marginalized.
Taylor draws attention to the importance of representation and privacy – that people have a right to privacy the same way they have the right to be represented within the right datasets. The engagement pillar advocates for the need for people to have the freedom to decide whether to engage with specific technologies. On the other hand, the non-discrimination pillar of data justice grants people the power to identify and challenge bias in the use of data, and the freedom not to be discriminated against.
Furthermore, data justice emphasizes the need to adhere to international human rights law, meaning that during the implementation of digital identity systems, it is crucial to ensure that digital personal information is safeguarded. Relevant articles of the UDHR and the International Covenant on Civil and Political Rights (ICCPR) guarantee all persons the right to privacy.
More importantly, it is worth noting that the datafication of society and the way data is generated, analyzed and used should not be viewed only in isolation and from a solely technological point of view, but within the context of the numerous social practices in society. Data justice plays a critical role in enabling us to situate concerns that relate to data practices in the broader context of social justice concerns.
The data justice framework also enables us to examine data in a very unique way, including its politics, power, ethics, and trust. Notwithstanding, due to the social injustices it seeks to tackle, the framework is significantly strengthened by embedding a human rights-based approach. By ensuring that human rights are at the center of the discourse, it ensures the data justice framework benefits from a global human development perspective – which is an aspect that data justice cannot independently do.
Section 4: Challenges in Implementation of Data Justice
Achieving data justice is quite challenging due to various inherent biases and complexities. The three major challenges are technological challenges, regulatory challenges, and international disparities.
Technological Challenges:
Systematic errors in a data model can contribute to incorrect predictions. Such errors can emerge from diverse sources, such as the choice of features used for developing the model, selection of training data or the algorithm utilized for training the model. The most common types of bias include measurement, selection, and algorithmic biases.
The selection bias occurs when the training data is unrepresentative of the population being modeled, resulting in biased predictions. Contrarily, algorithmic bias manifests when the algorithm utilized to train the model yields biased prediction because of inherent biases within the data or algorithm utilized to train it. Selection bias means that certain segments of the population are systematically underrepresented or excluded within the data sample, resulting in skewed results. It can be occasioned by factors such as nonresponse bias, demographic disparities, or sampling methodologies.
For algorithmic biases, when biases are embedded in machine learning models or algorithms, it unfairly discriminates the outcomes of certain groups. It can emanate from flawed algorithms, biased training data, or inherent biases within the decision-making process. On its part, measurement bias manifests when the measurements used for training the model are imprecise or inaccurate, resulting in biased estimates. This implies that measurement bias is caused by inconsistencies or inaccuracies in data collection instruments or methods, resulting in distorted assessments or measurements. The most common sources of measurement bias are linguistic or cultural differences, measurement errors, or subjective interpretations.
Data collection processes can also reflect social biases and historical prejudices. These exacerbate systemic inequalities and marginalize underrepresented groups. For instance, biased recruitment practices or discriminatory policies can unfairly represent demographic information in employment data. Data collection instruments and methods can also be biased. For example, questionnaires or surveys can contain language barriers, cultural biases, or leading questions that skew responses and distort outcomes.
Since human judgment and interpretation are important when collecting and analyzing data, they become vulnerable to cognitive distortions and subjective biases. Cognitive biases that can impact decision-making and analysis are anchoring bias, availability bias, and confirmation bias. These biases introduced complexities in technologies including inaccurate insights and decisions, reinforcement of inequality and stereotypes, and loss of credibility and trust. Biased data analysis can result in misleading or inaccurate insights, thus undermining the reliability and validity of decision-making processes and leading to missed opportunities and suboptimal outcomes.
In a nutshell, bias in data collection and analysis processes poses challenges and complexities to the decision making process’ fairness and integrity within the digital era . By understanding the impacts and sources of bias, and embracing mitigation strategies, organizations can promote equity, accountability, and transparency within data-driven initiatives, eventually instilling confidence and trust within the data ecosystem.
Regulatory Challenges and International Disparities
The complexity and fragmentation of data laws is a critical issue that businesses today face. One of the core challenges is compliance burdens. Ideally, businesses that operate across multiple jurisdictions should navigate diverse legal requirements that have conflicting and even overlapping provisions. This can be time-consuming and costly, requiring specialized expertise and adaptation of practices and policies.
Uncertainty and risk is another key challenge. The evolving or dynamic nature of regulations and likelihood of inconsistent interpretations by various authorities create uncertainty for businesses, thus aggravating the risk of penalties and non-compliance. Cross-border data flows are the other major challenge. Ideally, inconsistent requirements make it quite difficult for businesses to not only develop, but also deploy innovative services and technologies that depend on data sharing across borders.
To address these challenges, the potential mitigation strategies and solutions are convergence and harmonization efforts. Initiatives such as the Global Privacy Assembly and the APEC Cross-Border Privacy Rules Framework seek to enhance harmonization and convergence of data privacy laws across various regions. Secondly, it is crucial to develop standardized tools and frameworks for data governance and compliance to aid businesses navigate this complex landscape in a more effective way. Thirdly, emphasizing/focusing on adherence to core data principles such as individual control, accountability, and transparency can lay a foundation for compliance even within diverse regulatory environments.
Despite data being essential, its management usually poses detrimental challenges, especially when it becomes fragmented across various platforms and systems. This fragmentation can disrupt the compliance operations’ very essence, resulting in increased risks, higher operational costs, and inefficiencies. Data fragmentation is defined as the scattering of data pieces across multiple storage databases, locations or even varying formats.
Fragmented data can imply that critical information regarding a customer’s transaction history can be stored within one system, whereas risk assessments or ID verifications can be stored within another. The result is a complex web of data that makes tasks tedious and poses potential regulatory risks. Compliance data fragmentation – where crucial pieces of compliance information are scattered across different locations and systems – poses detrimental challenges. The core impacts of compliance data fragmentation are operational inefficiencies, increased risks, higher costs, impediments to innovation, and damage to reputation.
Conclusion
Data justice systems can help uncover biases based on gender and ethnic lines. Thus, the systems can ensure that data practices deter biases and advance data rights that empower marginalized groups while propagating social justice. This underlines the critical role of reducing biases and inequalities and promoting fairness and social inclusion for vulnerable populations.
The other finding is that data justice can ensure equitable access to data, thus enhancing data equity, health, and well-being, embedding fairness into algorithms, and promoting diversity within teams. Furthermore, data justice ensures that data management is transparent and accountable. In addition, data justice is effective in ensuring that organizations safeguard confidential and sensitive information.
Moreover, data justice effectively ensures algorithmic fairness, enhances participation and representation of all groups and individuals, and promotes non-discrimination/equality. To this end, a human-rights-based analysis is pivotal in advancing data justice. For optimal outcomes, the intersection between data justice and human rights must be emphasized.
Future policies should emphasize the intersection between data justice and human rights. Numerous vulnerable segments of society seek social protection through digital identity systems, which highlights the importance of data justice in shaping the future. This presents an opportunity for policymakers and authorities to reassess the existing frameworks based on the design of digital identity systems.
Technology experts should take into consideration how core socio-ethical challenges generated by AI systems recently have clarified that this technology significantly affects fundamental human rights. Also, stakeholders must adopt an integrated approach to enhancing data justice and protecting digital and human rights.